Getting Sensitive: Diagnostic Sensitivity and Specificity Simplified
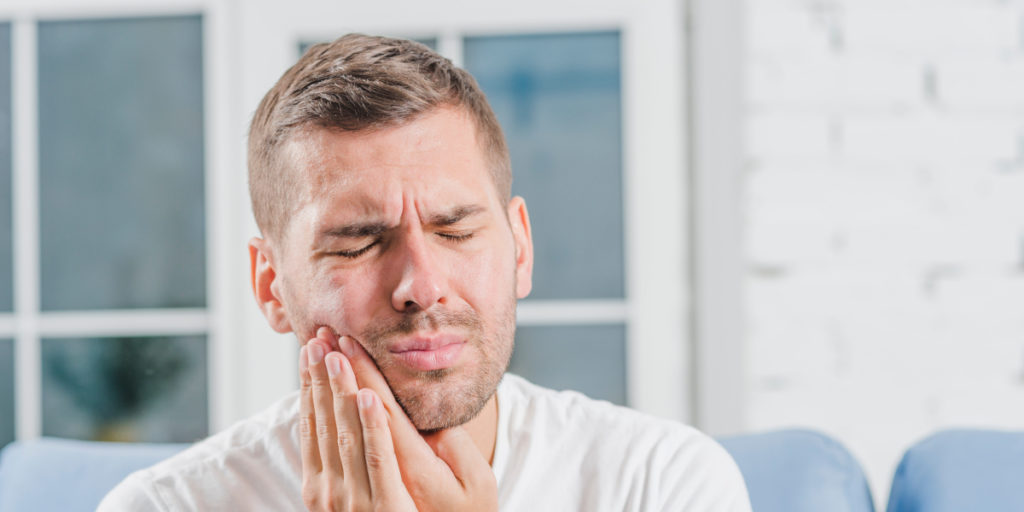
What Do We Mean by Diagnostic Sensitivity?
In clinical diagnostics, questions about the sensitivity of an assay will inevitably surface. But what does “sensitivity” mean exactly? The lowest quantity of the given analyte that an assay can detect is often called sensitivity – and to be clear, this quantity is the analytical sensitivity or Limit of Detection (LoD). The term analytical is key for that definition, so while we’re at it, let’s contrast that with the term diagnostic. Diagnostic sensitivity is related to the ability of one’s assay to correctly identify populations of individuals with the disease, and while this is certainly a function of analytical sensitivity, high analytical sensitivity (meaning you can detect very minute quantities of your analyte) does not necessarily guarantee useful diagnostic sensitivity.
As you can imagine, the two measurements are very different – the former telling you about the performance of your assay in the tube and the latter telling you about how your assay performs on a given population. For this reason, it is important to attach the terms analytical or diagnostic to the term sensitivity when describing your assay.
How Do You Calculate Diagnostic Sensitivity?
Another way to think about diagnostic sensitivity is to consider how well the assay can detect true positives. But if you are dealing with unknown samples, how do you know what is a true result? That is sort of a chicken and egg question, but let’s consider this.
Say you have an assay that can determine if a patient has five fingers or six on each hand. You can collect a sample, blind them to the experimenter and obtain a result. Next, have that same patient be examined by a clinician who would simply count the number of fingers on each hand. Then compare notes – for how many samples did your assay and the clinician’s observations match? The clinician’s observations in this case would be considered the gold standard since you can’t really get more objective than counting fingers! If the goal were to detect the six fingered individuals (i.e. six is a positive result), an assay result matching a count of six would be a true positive, while an assay result of five for a five-fingered patient would be a true negative. Likewise, an assay result of six for a five-fingered individual would be a false positive and an assay result of five for a six fingered patient would be a false negative. If we took the imaginary data set below, we could calculate diagnostic sensitivity by calculating the percentage of true positives detected out of the total actual positives in the samples (true positives plus the false negatives).
Patient No. | Observed No. Fingers | Assay result (No. Fingers) | True Positive | False Positive | True Negative | False Negative |
1 | 6 | 6 | X | |||
2 | 6 | 6 | X | |||
3 | 5 | 6 | X | |||
4 | 6 | 5 | X | |||
5 | 5 | 5 | X | |||
6 | 6 | 6 | X | |||
7 | 6 | 6 | X | |||
8 | 5 | 5 | X | |||
9 | 5 | 5 | X | |||
10 | 5 | 5 | X | |||
Totals | 4 | 1 | 4 | 1 |
The data above can be tabulated in the truth table below and used calculate the diagnostic sensitivity using the following equation. Here we are calculating the percentage of individuals who have the condition and have a test result that is positive for the condition.
True Condition | |||
Positive | Negative | ||
Condition predicted by assay | Positive | TP | FP |
Negative | FN | TN |
True Condition | |||
Positive | Negative | ||
Condition predicted by assay | Positive | 4 | 1 |
Negative | 1 | 4 |
Sensitivity = \frac{\mathrm{TP} }{\mathrm{TP+FN}} = \frac{\mathrm{4} }{\mathrm{4+1} } = 4/5 = 80\%
Not too bad, right? Shall we look at a real-world example? Imagine that you are developing a qPCR assay that detects a bacterial pathogen. Results obtained using your qPCR assay would yield data for the predicted condition and these would be compared to results obtained from classical culture. Why? In this example, recovery of the organism by culture from the diseased patient is one of Koch’s postulates and is why bacterial culture would be considered as the gold standard. If we had this contrived data set:
True Condition | |||
Positive | Negative | ||
Condition predicted by assay (i.e. qPCR positive) | Positive | 238 (TP) | 21 (FP) |
Negative | 2 (FN) | 103 (TN) |
We would calculate this diagnostic sensitivity as:
\frac{\mathrm{238} }{\mathrm{238+2}} = 238/240 = 0.992 × 100 = 99.2\%
That means if we were to employ this qPCR to test patients for this bacterial pathogen, we’d get the true positives right 99% of the time. But what about the false positives? You would indeed detect them too with this assay because a qPCR detects DNA from both viable and non-viable organisms, while culture would detect only viable organisms. Not to mention, qPCR is likely to have a much better analytical sensitivity than most culture-based methodologies. Comparing these two methods and assigning culture as the gold standard would define culture-negative/qPCR-positive samples as false positives. Under this scenario, you would probably want to run a confirmatory test just to make sure that a qPCR-positive patient was truly infected with a viable pathogen causing disease.
What About Diagnostic Specificity?
While the number of false positives in the above example might worry you, the real judge of performance depends on how your assay is being used. If your goal is to rule out healthy patients to avoid confirmatory testing, then a high diagnostic specificity, would be key. Oh wait, I just introduced another term – diagnostic specificity! This is a related measurement of how likely your test is to correctly identify those individuals without the disease. Think identifying the five fingered patients correctly, or detecting those patients not infected with the bacterial pathogen. Here we are calculating the percentage of individuals without the condition and correctly test negative for the condition. That calculation follows:
Diagnostic\; specificity = \frac{\mathrm{TN} }{\mathrm{TN+FP}} = \frac{\mathrm{103} }{\mathrm{103+21}} = 103/124 = 0.831 × 100 = 83.1\%
That means we would correctly identify the healthy patients 83% of the time. Since the qPCR test is much more rapid than waiting for bacteria to grow, running the qPCR would be of benefit and you could be confident in the qPCR negative results given that we had few false negatives in this contrived data set. Any positive patients should of course be tested again using culture, but there would be fewer patients to test. You also use these calculations to compare a new qPCR assay to one currently in use or a qPCR to an ELISA. And if math isn’t your thing, there are a range of free on-line calculators, such as this one from medcalc, to run these numbers for you!